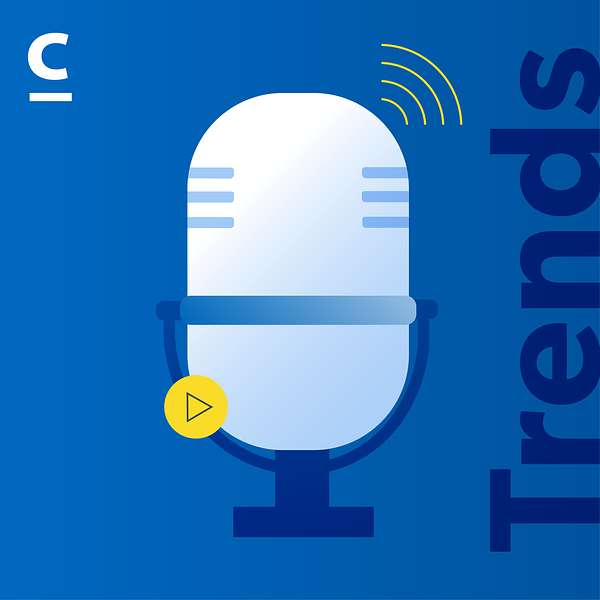
CapTech Trends
CapTech Trends features thought leaders and subject matter experts discussing emerging technology, design, and project methodology. Our goal is to unite diverse skills and perspectives to show how data, systems, and ingenuity can transform and enable organizations to advance what’s possible in a changing world.
CapTech Trends
The Latest in Actionable Data
In this episode, CapTech Technical Director, Cameron Snapp joins Vinnie to dig deeper into data transformation, the importance of data-sharing, and what’s new in the landscape.
Listen in as we dig into:
- Why understanding your data sources and communicating what happens to them, is important for the future.
- How third-party tools that sit in your data pipelines can make it easier to retrieve the data you need.
- The value of data democratization across an organization.
- Observing the shift of responsibility from building pipelines to now educating about them.
Vinnie:
Welcome back to the CapTech Podcast. We're going to go deep into data today, look at some data trends, I have with me Cameron Snapp, a technical director out of our Richmond office. Why don't you say hello, Cameron?
Cameron:
Hey, Vinnie, great to be here. I've spent the last 16 years with CapTech studying data architecture, Azure, SQL databases, so I've seen a lot of data transformations for a lot of organizations and I'm excited to talk about what's new in the landscape today.
Vinnie:
Yeah, I wanted to chat with you because the intent of data hasn't changed much if at all over time. It's getting actionable insights that the business can make sound business decisions on. And then when you start looking forward into machine learning and AI, it's being more predictive with the data. So there have been shifts in terms of the value of data and they seem to come in chunks. We have a couple new toys to play with and then eight years later there's a couple new toys to play with. So what's changing now that people should be aware of and where should we be looking for that next big change for an organization?
Cameron:
Right. Well, there's definitely always been the movement that we want to get good data, turn it into information and get into business leaders' hands. So if we're not doing that as our focal driving point, then that's really where we need to ahead. We're doing something wrong if we're not thinking around actionable information for business leaders to make better decisions.
Another piece of it is that data governance is something that everybody likes to talk about. It's a key for information management, but with recent changes around privacy in the CCPA, California Consumer Privacy Act in the United States, and GDPR, General Data Protection Regulation in Europe, and the recent LGPD, General Data Protection Law of Brazil, it's now actually legally mandated to be critically thinking about how you operationalize data and how you protect people's information.
So two things we really want to focus on always is data protection and good stewardship as well as making sure the data's meaningful and the information's useful.
Vinnie:
So let's dig into a couple of those. Because you said a lot. We could spend an hour on that, which we won't.
But data governance. Conceptually, I think most people can assume what that means. How would you describe what data governance means?
Cameron:
There's a lot of academic definitions around the pillars, but really it's about understanding the source of your data and being able to trace its' lineage through any processing you do and how it became a piece of information, an analytic device. It made its' way into a machine learning product. And then being able to communicate to all your users, "This is what happens," so that when they say, "Revenue is X or revenue is $1000," that everybody understands the same definitions. So we have one source of truth and we have one way that we think about that meaning of that data. So it's a lot about cataloging and making sure data literacy is another way to think about it, that it's secure and that it's accurate and protected.
Vinnie:
Well, it sounds like there's two sides to that. I don't want to go too deep into data governance, but I think it's an interesting place to start. One would be, technically, as data goes through when you're saying we understand what's happened to it. Is that all through meta-information, tagging of that data so it feels associated with that data?
Cameron:
That's a key component. So when we start thinking about exposing data for consumers, and one of the big trends we're going to talk about today is the different ways in which data's getting shared and exposed by different organizations.
But yes, tagging it to know where it came from and what its' business purpose really is meant to be. The more descriptions you can put on data, the more valuable the user will find it.
Vinnie:
And that's become more tool-based.
Cameron:
Definitely more tool based. But as we're seeing, too, being able to crawl the data metadata and there's actually some AI that's coming forward to say it can automatically detect when data might change and when data gets out of profile and so it can alert our data stewards to when it's falling out of compliance. And that's actually really interesting.
So master data management was always the tool, but now there's even more tools that do the monitoring and to make sure that what you cataloged and tagged is still true because we want to make sure we track with large data sets when something gets outside the norms.
Vinnie:
So I said there was two concerns here. The second is the people and process side of it. So you can tag data, but I forget how you exactly you phrased it, but that people know how to access and use. How do they know how to access and use?
Cameron:
Right. So the big movement and one of my major trends is around data literacy and the training. So as technologists in this space, I think we spent a lot of our careers worried about the nuts and bolts, the ones and zeros of coding. And I think our job now in the data space is being better communicators about what information is. So now we're turning from folks that build pipelines to folks that explain what pipelines do to our business users so that they have more empowerment and that they're actually understanding. And we're doing a lot more teaching than I think we used to. So we're raising that awareness of this is what data is and this is how you should use it and this is the value it can provide.
Vinnie:
So I've heard a term, and I wonder if this plays into what you're saying, data democratization. Is that related to what you just described?
Cameron:
Yeah, that's a huge trend right now. And it's really removing the barrier of technology and making it easier for any user to access the information they need to do their job. And so what traditionally used to be, "Hey, there's this data source, we want to bring it into our data warehouse and we want to put a really cool report on it so we can give that to Vinnie so that he can monitor X thing about our systems or our sales." That would be something IT would take. They would go into a box for a year and they would build all this stuff to generate that report.
Now that's not exactly the most scalable or valuable way to spend our time. And so what we're trying to do is get data into those people's hands. And then even BI tools, there's a bunch of no-code BI tools now. So technology's no longer necessary. They can get some training and they can start using the data and feeling that they have the right access that they need at the right time. And the faster we can get it to information and meaning is for everybody's benefit.
Vinnie:
Yeah. So now I want to touch on the technical side of what you're talking about. We talked earlier about data fabric and data mesh. And I wanted to touch a bit on the data mesh side because we were just talking about getting access to usable data and I have some thoughts on that. But before we get into it, can you talk about the differences between what a data fabric is and data mesh is? Because I hear them used almost interchangeably, but I don't know if that's by people who don't know the difference.
Cameron:
No, I think that's fair. And I think there are... Just Google that and you'll find tons of articles trying to explain this difference. So what my understanding, and first and foremost, these are concepts. These are not really tools. There are tools that do data fabric, but these are ways to architect your systems to be able to make the data available.
And so fabric you can think as a centralized body. So this would be a platform. There are multiple tools that do this. Atlan, Cinchy, data.world, Denodo, TIBCO, all have products, and not recommending any of those, just saying those are examples that are out in the space. And your organization would actually adopt one of these platform tools. But it makes APIs tap into all the databases and make it more widely available so no matter who you are, as long as you have access to the platform, you get access to all the underlying data. It's not a onesie, twosie of, "Oh, I need a username and a password to get to this SQL database on-prem," or, "I need my own Salesforce login to go access a bunch of the sales records."
And with the abundance of SASS tools and the abundance of different data platforms that are happening with COTS products, it got unattainable to get people the right stuff. So data fabric's a way to you can approach this. It is still, like I said, managed by a centralized bodies. So think about it that there's still an organization that decides what data we're going to make available and to whom and to how that data will be made. So it's still a little bit black box and it's still a little IT driven. But a good stat on this is that Gartner says that 70% reduction in data management tasks will be realized by the adopting of a data fabric.
To me it's a lot like data virtualization, which was a buzzword maybe five years ago, and now some of the products are using blending that as well. But virtualization's really around an abstraction layer that hides the underlying hardware, but it makes holistic access. So fabric is similar to virtualization.
Mesh is more conceptually domain driven. So different business users are deciding what data they want to make as a product available and they do it however they want. So it's a very decentralized way to do the same thing but the organizational change that happens and the physics of what's taking place is very different between the two.
Vinnie:
So that means that if I've got seven different business units, some might be using Snowflake and the cloud, some might have SQL server still On-Prem, some might be having a no sequel binary large object kind of data set that they... And so you're saying there's no centralized enforcement of how they're storing their data. Now are there guidelines here? I mean does IT have a role here to say, "This is the pallet of tools you should be choosing from?"
Cameron:
Absolutely. And that is exactly the risk, I think, that mesh brings is you're doing a great thing by empowering business units to have more control over their data and they get to decide how they're going to use the tools. IT becomes more of a governance board in this way and saying, "These are the best practices we want you to use and these are the policies that you have to adhere to in order to make this work."
Vinnie:
The first thing I think of, sorry to interrupt I got excited was done well, then. What I mean by done well is at the business unit level, having the right people there to size these tools and architect these tools correctly. You are taking a lot of these stage skating away from IT so that, instead of having a team that has 12 people who can do this, you now have five different business units each with their own team. So you could do more things in parallel and IT just becomes the body that makes sure it's done correctly-
Cameron:
Hopefully.
Vinnie:
... as opposed to the one doing it all.
Cameron:
Right. It definitely creates more agility and it also encourages collaboration. So the data engineers maybe for your sales domain would hopefully work with some of the folks in the HR domain to do similar type things, but they actually have less responsibility. They're not necessarily having to build a broad enterprise data strategy. They're working on the stuff they know. And so by having that education and background in the sales data and the comfort with it, I think they're able to productize and monetize their data much faster.
The key for any of this is that you really want to be thinking about data as a first class citizen and that you really have to have strong buy-in for governance and transparency and rules. Otherwise it's all going to fall apart. My fear is that domains will become little fiefdoms and they'll adopt these tools and then do some things outside the norms. There's also the cost side. Is it to anybody's benefit to license, you mentioned Snowflake, license Snowflake amongst a bunch of different domains. Or should there be one enterprise license for everybody's data is in together?
Vinnie:
I mean, I don't want to put a negative spin on it, but it's almost like empowering shadow IT. Right?
Cameron:
Could be.
Vinnie:
Because you're going to have basically small data groups, which could be data IT groups, across multiple domains each, like you said, fiefdoms only worrying about their own specific data set. That feels shadow IT-ish, but I guess if, like I said before, if you do it well and it's has the right oversight from IT and the right guidelines from IT, then I guess it's more federated than it is shadow.
Cameron:
It's definitely more federated. I think it's also, we saw a real big movement towards getting analysts ad hoc power BI-type dashboard tools. So they wanted their hands on their data, they didn't want to wait for it to build those things I was describing earlier on. And so I think this is the next evolution of that. That now the data analysts are super comfortable knowing what they want to do, they're technical savvy and so they have a broader access of tools and more analytic. They might be running Python, they might be running R, but they're really comfortable and they understand their data and they want to tell a story with it.
So the visualization tools are becoming a part of that organization. And I think as more and more folks see that adapting dashboards into your PowerPoint presentations is now an afterthought, people just assume that's going to be part of their data literacy.
So I think that movement away from the bottleneck of traditional IT waterfall projects to getting these data domains up and running and in parallel is really creating efficiencies and creating a better customer experience because there's just a lot more thoughtfulness around what the story the data is telling and it's more accurate.
Vinnie:
So I'm going to expose a bias to you. I grew up as a developer. I started programming when I was 12 and just did that early part, mid part of my career, and got into services development and APIs and abstraction is a very common theme in application development and coding. And I feel like data, and of course I had to know a lot about data because back then you had to do a lot of your own wiring of these things, seems to be lagging a little bit behind application development from an engineering maturity standpoint, but not by too far. And what I mean by that and probably a bunch of data people just drove their cars off the road yelling at me.
What I mean by that is, you had DevOps and then of the data equivalent of that was a fast follower to that. And when I hear what we're saying here, we have decentralized data and we are basically creating an abstraction that creates a model of different types of things in our organization. That to me sounds like web services, APIs.
Cameron:
Sure.
Vinnie:
That we would write in front of data or other services as an abstraction that would allow anyone to access things without knowing the detail behind it. So when I hear you talk about data mesh, I start thinking, "Well isn't this just another way of creating API endpoints?"
Cameron:
Yeah. And I think that's a great call out that whether or not you do data mesh or data fabric and you get buzzy with that, I think the way you do data services and data exchange is certainly much more of an object-oriented approach, much more of an abstraction approach. And so while I wanted to talk about the trends, and I'm seeing that fabric and mesh are becoming more of a thing. This is something I think everybody should be putting more diligence into is thinking of data as a product and your data systems with CICD, with good code repositories. And we are catching up. I definitely agree that 10 years ago there was really poor performance on deploying data schema changes and things like that. So just having code commits and branching in database tools is more common now. So I think from that aspect, we have caught up and we're doing better.
But I think data engineers have now become software engineers. We have to know how to build API endpoints. We have to know how to interact and source data from a large variety of things. We're not just touching ODBC connections to Oracle SQL Server and a flat file off a shared drive. I just think the idea of external data coming in is a huge thing. And then being able to allow your data to be consumed outside your organization more broadly is a huge trend as well.
Vinnie:
It would seem, well, it feels like then that the skill sets are changing.
Cameron:
Skill sets are evolving a little bit, I think.
Vinnie:
Okay, fair. Yeah.
Cameron:
So we still need to have really good best practices on frameworks and the way we do repeatable code and way we think about things being maintainable. So I've always felt as a ETL developer, so cutting my teeth on SSIS or Informatica back in the day you thought about data movement, but you had to put in robust row counts and air handling and good stewardship of the process. And so I think that is still core and fundamental. So we can talk about data engineering tools in a little bit, but I think the idea of how to create something like a piece of software and maintain it over time is still very much important.
Vinnie:
Well let's go ahead and jump to the tools because I know I oversimplified when I said, "The mesh is an abstraction tier similar to an API," because I know it does some more advanced analytic-type work for you and it's more advanced than that. So I wanted to make sure I didn't... You made me make a loose analogy and move on. So why don't you jump into the tools a bit and tell us a bit more about that.
Cameron:
So there's an abundance of engineering tools, and this is... So I was talking about some of my collaborators in the data engineering space here at CapTech, and this was just such a hot topic. Third party, no or low code tools are just everywhere. And so specifically I'm talking about things you license like a Matillion or a Fivetran, a DBT, which is open source or a Databricks live tables that if you're using the Databricks platform is a way to build pipelines.
And so all of these are periphery useful to your cloud provider standards. So Azure Data Factory, AWS Glue, Google Fusion, those are your more traditional data orchestration. These other tools kind of sit inside of those pipelines or augment those pipelines. This is a much easier way to connect to a broad set of sources. So they have built in connectors, they're making it easier to just get the data that you want and bring it in.
So you have to be able to write good SQL and Python, but otherwise leave all the plumbing to the vendor. And so this is something that, again, the evolution of the data engineer, we have to do less data connectivity and more data processing, profiling and stewardship. So those are some specific tools. And then in the warehousing slash analytics space, there is Redshift, BigQuery, Snowflake and Synapse. Those are all data warehouse tools. You don't want all of them. You have to kind of pick. And so just navigating that alone is something that's really difficult for clients that we see on a daily basis.
Vinnie:
Gotcha. So staying away from our clients, who in the market is known for doing this well? Who using these modern tools and having good results? Are there general case studies that are out there?
Cameron:
Sure, there's a really good case study that's pretty prevalent on Netflix with data mesh. So if that's something you want to learn more about, that one I think's pretty easy to find on Google.
As far as data exchange, there's a couple good ones. A lot of it came, some of them came out of the pandemic. The idea of data sharing became immediately necessary. And so a bunch of different health organizations and state agencies had to figure out how to share data more accurately, broadly, and people needed it in pretty real time. So I think crisis aversion in the healthcare space and patient outreach. I think another good use case for this would be how do we decide who needs to get vaccinated when and where? So we actually saw a lot of healthcare providers, the big insurance companies working better with pharmacies and local state organizations to get that data into the right hands of people so they could figure out where and when to hold vaccination.
Vinnie:
But why is it easier? Why is it better?
Cameron:
Because they've decided that we don't need to just have the data on our side and let them figure it out. We're going to expose that data out into the environment. And so that B2C or B2B business-to-business, business-to-customer way to interchange data that we kind of were teeing up with these data services, it's now actually critically important to these movements that are socially necessary and also business necessary.
Vinnie:
And that goes back to a phrase you used early on, but it was kind of buried, that data productization. You're making this sound much more like data as a product.
Cameron:
So we started the conversation around how to get information into business leaders' hands. And that's still really important. But the idea now is also that data is useful to other organizations and other entities. So an example there would be that Uber and Waze were sharing some traffic data that they had that was proprietary to their system, they didn't have to make it available, but they made it available to the Department of Transportation to say, "Here's where bottlenecks are happening. If you can address this, our customers will be happier and your citizens will be happier." So that's another use case of just something outside the box that we're seeing on data sharing and by making... If you build the right infrastructure, and this goes back to just use the right tools, steward your data the right way, put data services on top of your data to make it available and abstracting it from your system. So you're still doing good protection, but now you open the door to the impact your data can have outside of your organization.
So I like to encourage clients and any companies, anyone listening, don't just think about how your company needs to use data, it's how can other people and your customers have better access to the data.
Vinnie:
And how could you benefit from other people's data? Look bidirectionally.
Cameron:
So this is a huge one too, is that everybody wants to get into machine learning and AI, but you have bias in your organization data. If you only have your customers, you only know about them. And so if you can go out to the marketplace and get broad customer data, you can better train your AI models.
Vinnie:
I would say that slightly differently. If you only have data on your customers, then you don't have data on-
Cameron:
The potential customers.
Vinnie:
... the potential customers.
Cameron:
Right, right.
Vinnie:
The people like why don't you have them, right? I think that's important.
Cameron:
So you brought up Snowflake earlier, one of the cool things they've done with the marketplace is that anybody using their tools can make their data sets available to other Snowflake customers. And so there's a little bit of a data exchange going on now. So again, just thinking about a fact and dimension model that you would use to build reports. It's a huge evolution to now say, "I'm going to expose this protected… obfuscated, but still customer data out," and other people can absorb it and use it for hopefully good, but to be able to create more robust AI systems.
Vinnie:
So I know data accessibility and data ethics are gaining mindshare in terms of what's important in an organization from a data perspective. Is there any tooling? The modernization of these tools, are they helping in those ways or is it just a process?
Cameron:
I think it's an evolutionary process. This is the people side of the lens that we really want to be thinking about how to make sure the people are moving forward with the technology. We don't want to just make a bunch of data available and not teach anybody that's there. So a good resource for this, it's not a tool, but it's a website called the Data Literacy Project. Dataliteracyproject.org. And there's a slash human impact. There's a really good article about how to think thoughtfully about democratizing your data and putting it out there.
So the thing with data ethics is more just a mindset that I think we all have to have if you think about the headlines right now around social media sites and their data usage, your mobile phone tracking, your data, and the data breaches that happen to any number of corporate entities, data is very risky and you can ruin your brand.
And customers have an expectation nowadays that they have a right to privacy and they have a right to know what you have about them. So if you're not putting systems in place to allow customers to have that view and that you're thinking of them as this outside actor, I think you're setting yourself up for trouble. So the ethics side of this is customer privacy is an expectation and a right and you have to address it.
And so when you think about new features you want to roll out, make sure you're rolling out the feature to the right user and not doing something that might harm them or they might not like. And then there's also, we talk about the tech side of ethics, there's a movement for blockchain, based chain of custody on data lineage. So there's ways we can leverage these more modern, buzzy tools to make sure that we're being ethical and thoughtful about the way we're sharing and using data.
Vinnie:
Yeah, look, it's a good trend, but some of it can be annoying too. So now because of all these laws, every time I go to a different website, before I can enjoy the website or learn what I need to learn, I've got to go in and set up all my cookie preferences on every site as opposed to just putting it in my browser settings that these are the ones I want and don't want. Because it could be, even if you did that, you would lose some flexibility because you do want different things from different places. And I know that there are people out there, organizations out there that are creating products and services to help with this, but we're sort of in the pain curve.
Cameron:
We're very much in the pain curve. So you and I are both guitar players, and this is an exact use case I thought about the other day was a band I liked was advertising that they did a YouTube video that they showed their rig rundown. So I got to see a pedal and I was like, "Oh, that pedal seems pretty cool. I'm going to go to the corporate website and read about it." And then I was like, "Oh, I want to read some reviews on third party sites and I'm going to go to a retailer and see what that pedal is." And I was like, "Cool, I learned something today, I don't want to buy it. But I enjoyed that." Now my browser is nothing but popups from all those sites about all the pedals I should buy. And so that's a negative user experience for me where I'm just trying to learn more and the fact that they have all that data about me now is just almost unpleasant and it makes me hesitate to go to those sites anymore. So I think there's something there too.
Vinnie:
At least yours is guitar related. I clicked on a link, Matthew Perry from Friends wrote a book on his addiction and it came up in a feed, I clicked it, thought it was interesting, and now I'm just inundated with Friends links all... It just...
Cameron:
I guess that's better than opioid things too, but...
Vinnie:
Sure. Absolutely. So I want to wrap up, but before we do, I want to have you address how do people get started? So people listening to this, they get excited about this, they want to know what their first couple steps are. What do you do the first three months? So you listen to this podcast, you want to get more. Help me out.
Cameron:
I think you have to put some thought into a data-governance role at your organization if you don't have that. So depending on your size, somebody should be taking on responsibilities of a chief data officer to be the person who's very thoughtful about the way data gets used. And they should craft some policies and some... Before you go to tools and mesh architecture. If you don't have somebody advocating for the rights and the integrity of data, you're going to be in a bad place.
So then I would be thinking about just cataloging all your sources, understand where in your ecosystem data is important, and then think about your consumption. So I always like to do left side, right side of the diagram. Where does data come in, how do we store it in the middle and how do we let it go back out? So we see a lot of companies do an okay job with documentation, but I think it's really a good time to inventory how you want to process data. And then you can say, "I'm ready to bring in this new tool or this new mindset or this new capability in the cloud," to start heading down the road to better information maturity. So it's very much a crawl, walk, run approach. You can't just launch into a brand new architecture. You have to be thoughtful about it.
Vinnie:
I would add one thing to that, because I don't disagree with anything you said, but if I wanted to get adoption, as I'm thinking about that data-governance role, I would be meeting with the business before any of this and saying, "What can you do with the data you have now? What do you wish you could do? Wouldn't it be great if you could? Do you know other people are able to do this?" And really start getting the mental buy-in and excitement from the business because then everything that you said becomes much more supported and easier to execute on.
Cameron:
Right. It's all about a plan for a data-centric organization. So you brought up the what can you do and what can't you do. Ask people what keeps them up at night. What scares them about the data? Where are the biggest hurdles or challenges to their everyday job and tackle those. Because if you have quick wins and a huge return, sometimes it is just good documentation and policy. It doesn't involve any tools or licenses at all.
But on the flip side, I think looking more at what tools are in the marketplace, we're kind of getting to a point that we're looking at the transactional data more in its' raw format, so any tool that's going to allow for better data exploration quickly, and then also figuring out whether real time data processing is right for you, but be real about what your needs are. Do you need data up to the second, up to the minute just from yesterday? So when you're talking about who your business users are, like ask that question. How timely are the decisions they're making with the data that's coming in? Because that completely changes your architecture and it changes the level of severity of your risk tolerance to how soon the data can make it in and what does a support model look like.
Vinnie:
Great. Well, thanks for coming on the podcast. Very much appreciate it.
Cameron:
That was awesome.
Vinnie:
Yeah, great talking to you on this stuff. And for the audience, thanks again for listening in and expecting a new one out pretty soon.
The entire contents in designing this podcast are the property of CapTech or used by CapTech with permission and are protected under U.S. and International copyright and trademark laws. Users of this podcast may save and use information contained in it only for personal or other non-commercial educational purposes. No other uses of this podcast may be made without CapTech’ s prior written permission. CapTech makes no warranty, guarantee, or representation as to the accuracy or sufficiency of the information featured in this podcast. The information opinions and recommendations presented in it are for general information only. And any reliance on the information provided in it is done at your own risk. CapTech. makes no warranty that this podcast or the server that makes it available is free of viruses, worms, or other elements or codes that manifest contaminating or destructive properties. CapTech expressly disclaims any and all liability or responsibility for any direct, indirect, incidental, or any other damages arising out of any use of, or reference to, reliance on, or inability to use this podcast or the information presented in it.