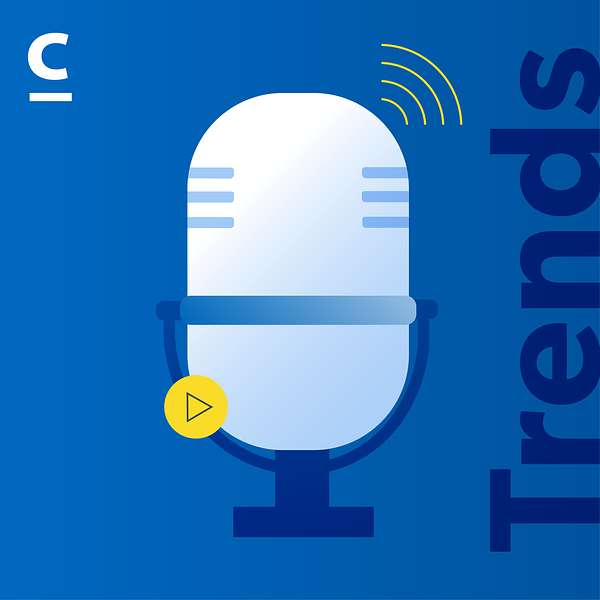
CapTech Trends
CapTech Trends features thought leaders and subject matter experts discussing emerging technology, design, and project methodology. Our goal is to unite diverse skills and perspectives to show how data, systems, and ingenuity can transform and enable organizations to advance what’s possible in a changing world.
CapTech Trends
How Trusted Data Pays You Back
In this episode of CapTech Trends, we dig deeper into our 2022 Tech Trends with data expert Michael Fitchko. This episode is rich with case studies and advice on how to grow your data productization efforts, whether you're just starting or have a mature architecture.
Listen to learn more about:
- Why the relationship between your data and your company's vision is important to success.
- Getting started with trusted data, not perfect data.
- Shift from looking forward, not backward, with mature data.
- Two case studies: one on how data cleansing led to an improved user experience, and another on how data productization prevented employee attrition.
Vinnie
Welcome back, everyone to the CapTech Trends Podcast. Today we're hitting data productization for a second time with a few more case studies and ways to get traction. I have with me Michael Fitchko, a director out of our Reston office, Michael has a lot of direct experience and he's a thought leader in the data space. Michael, welcome.
Michael
Thanks for having me Vinnie. Great to be here.
Vinnie
Let's get started with the phrase data productization. I struggle a bit with buzzwords or buzz phrases, industry terms because I feel like sometimes, they are overgeneralized and get kind of white papery. I kind of like the details behind there. I think when a lot of people hear data productization they think of data monetization. Is that what we are talking about specifically, or that and something else?
Michael
In part, right? I mean, data monetization is an important aspect of data productization as a whole but productization is really commoditizing your data to add value. So, however, your organization defines value, that's what you're trying to get out of your latent data assets.
Vinnie
Let's get specific on that. On the monetization side, which means we have data that's insightful, interesting, meaningful, and valuable to other people that we can sell directly.
Michael
Yes.
Vinnie
It also means perhaps we maintain that intellectual property and expose an API and charge for the interaction of that data.
Michael
Yep, exactly.
Vinnie
It could also mean internally, understanding what features assets of applications and services should be prioritized for use cases.
Michael
Correct.
Vinnie
Could be personalization too, right?
Michael
You are hitting it. Personalization is really, you could boil almost every data productization thing down to personalization. Yeah, you're hitting it on the head. That is exactly right. I think it is defining the monetary value of your data assets, or your intellectual property, acting against your data, turning data into information, but also being able to execute against that. I think that is the differentiator with data productization over something like data governance, right, where you're taking that next step to actually recognize the value of your data.
Vinnie
Right on an earlier podcast, I spoke with Arjun, and we went a bit deeper on that on what a Chief Data Officer role is, their responsibility, and organization, as opposed and really valuing data as the true asset as it is, as opposed to thinking only as a necessary back-office compliance thing we have to do. We won’t go too deep into that, there is another podcast on that show. Specifically, I wanted to talk to you about what our clients are seeing. When you go into accounts, and people are hearing data productization, and they're coming at you with, I guess, an end state in mind, how do we help them get there? What are some common gaps? Some common successes? What are you seeing?
Michael
Well, even backing it up. I mean, we're lucky if we go to a client, and they have a clear idea of what the product is they want to build. I think, because it's so new, and we get into the data productization assessment, people expect us to have a menu and you get to pick and you know, we'll just give this to you. That's not necessarily the case, right? It all starts with realizing or defining your company's value proposition and then trying to take action against that value, leveraging your data and improve the value proposition or create net new revenue streams.
Vinnie
We see this a lot. We've seen it with mobile computing before and web computing before that, and IoT, it's tying the solution to some corporate objectives, some corporate vision, right? A vision is not a strategy. Right? When I go in to do strategy work, I look for what that high-level vision is, and then what strategies are in place across the different businesses to get there, then I think of what audiences need to be targeted to achieve that strategy. Then I get into what needs to be built for this audience from an experience stance. So, we've been thinking about sort of that lifecycle from application development for a long time, but not data. I think that's one of the key things if people still are viewing data differently from an asset perspective than they are applications and shared services. Yeah so, give me some examples. Give me one monetization example. One non-monetization example. We can kind of drill into those.
Michael
Sure, yeah, we'll start with the monetization example because I think that's a little more exciting. A couple of years ago, worked at a client that was a sophisticated high-volume marketer, so consumer base of 30 million at the time and executing thousands of marketing campaigns a day against each consumer. So do the math in your head. That's a lot of transactions a day, a lot of data being created, and a lot of things to keep track of.
Vinnie
So, we're talking volume and velocity?
Michael
Yes. So, with all that there was a lot of customization in place already. If they knew your name, they put your name on the marketing campaign so that it felt personalized and goes back to that personalized messaging space. They sourced and validated your name from hundreds of different sources. They leveraged a third-party algorithm, a very well-known algorithm that a lot of companies use to do this type of activity, kind of “know your customer” stuff. To say, I have 20 different versions of Mike Fitchko name, lets you use the algorithm to validate which one he prefers. Say this is this customer's name. So, along the line there a lot of business process. Occasionally, there'd be some bad actors mid process that would change a customer's name to a profane word, right? Imagine you're rude to someone anywhere along the process, any number of reasons as to what happened, like your friend got into your account playing a joke on you, that was 90% of the use cases was people playing jokes on each other. Either way, it resulted in a degraded user experience, because now instead of Michael is my first name, it would be a very bad word. So, all the emails, mailers, phone calls, text messages, all of it.
Vinnie
And as funny as that might be. It's a horrible user experience and you would expect the company to not let that slide, right?
Michael
Right, a company with that large of a consumer base, you'd think would have their stuff together, right, which they did tremendously, however, it was very hard to track. That resulted in integrated customer experience and potential litigation. It's a big fish in a big pond and if you did something bad to me that they were worried about litigation left, right, and center, that was a huge cost savings for them to prevent litigation. So that was a big driver for this that got it off the ground. We found out that the algorithm they were paying for had a very basic profanity filter.
Vinnie
Basically, list and word matching?
Michael
Yeah, it had to be the whole word, very simple list, and nothing in between. So, it caught some stuff, but not enough. What we did was, implemented a regular expression to look at the patterns of the words themselves to find kind of hidden or latent, or extremely similar.
Vinnie
Using funny characters to replace common characters.
Michael
Yeah, exactly like that. In addition to some web scraping of publicly available kind of slang resources, like an Urban Dictionary, things like that to pull in modern, relevant, profane words that could exist.
Vinnie
I would imagine that's a model that can adapt over time as well because things change.
Michael
Yeah, absolutely.
Michael
Yep. So, it was definitely low maintenance. That was one of the requests or requirements for this project. We don't want someone's full time job maintaining a list of profanity, so certainly had to be dynamic and leverage kind of low code solution to keep it up to date and dynamic.
Vinnie
What gets me as I think about, my background is more application development, working with data as a back end when I needed, you know, persistence model, whether it be sequel or no sequel or whatever. The way I would address something like this is pattern matching, regular expression, the scraping gets a little bit more interesting. Those seem like common application development approaches, what I think gets lost in that detail, when I think about it from a purely app dev perspective, is the volume and velocity. There's a tremendous amount of data every day that needs to be updated. Really, really, really, really quickly. So, not to say that the process was easy. I'm saying it's easily understood. I knew what the problems you had to solve but I don't necessarily understand how you solve it at that scale and that velocity. I guess that's where a lot of the more modern tools come in.
Michael
Yeah, absolutely. Back then they were paying for brute force processing time to not affect the SLAs, the marketing campaigns. But with today's technology, with your Azure, AWS, GCP offerings, you're still paying for it, but it's scaled so quickly, that the infrastructure behind the scenes to do that level of processing in that amount of time is a lot more attainable, right? You don't have to be a multi-billion-dollar company to afford it.
Vinnie
Help me out. This sounds like a good case study for a data cleansing podcast. How does this relate to monetization?
Michael
Yeah, the output of that whole solution was this clean list or reference list, right? That plugged in very well to that algorithm and similar algorithms that a lot of competitors or related companies with a shared customer base, probably are facing the same issue. So someone along the lines had a good idea of, we know, this is probably a shared pain point across the industry, let's set up a subscription model so that folks can either pay once for this list, like every six months where they can afford it, or subscribe to the API, such that every time we do the scrape and update the list significantly, it's pushed out to subscribers, right? So, they can leverage that data pipeline to cleanse their data and improve the customer experience or however, they're going to use it across the board. The list, the byproduct of this was that net new revenue stream, because they identified the opportunity to provide uber cleanse data up to the day. That's kind of the differentiator between what was a mitigative solution to net new revenue stream leveraging a data product, so the output that list was the product itself and that became a monetized asset, leveraging existing data in existing business processes.
Vinnie
This makes me think of two things. One, it's a phrase I use quite a bit. Its unexpected dividends is the phrase, right? If you architect something well, and you followed design patterns, application design patterns, enterprise design pattern, data design patterns, you get unexpected dividends down the road. It pays you back. If you do point solutions, you get expected problems. It's just so much easier to do it right the first time. It reminds me of Amazon when they were architecting their solution, and the Jeff Bezos memo of, I think was 10 points or something about how the systems will be built. That created not only an excellent system for them, but then they did exactly what you're saying your client did. They took that model and externalized it created their whole cloud out offer out of doing their system correctly. That's a perfect example. I mean, I think it's a really strong analogy for this company doing what they needed to do but having a bigger view of the value that not only would that be to them, but to other people.
Michael
Yeah. And that goes back to what we said at the top of the podcast, define the value, understanding the value of the data is the crux of data productization.
Vinnie
Auto manufacturers, and I'm trying to get more data on this, but they're reporting that they're some auto manufacturers are reporting that the data they're getting off of their customers and their customer vehicles are more valuable than the revenue they're making from the sale of the vehicle.
Michael
Oh, yeah. 100%. I can see that happening. That goes into the trusted data source aspect of it. If you're talking about, what do I need to get started in data productization? Trusted data sources, number one, start there and see what we can do with that data. I tremendously, completely agree with that. I see that being very much reality.
Vinnie
Well, that's going to come into an ethics and legal situation. If I buy a vehicle from you, why do you own the data of how I'm using it? It can get tricky.
Michael
Personal view is I should be compensated for the data you're using against me, but I think that's a different podcast. (laughing)
Vinnie
I think we have had that podcast. (laughs)
Michael
That's another data product there too.
Vinnie
We had a MePrism podcast on that. If anybody wants to look that up. So, give me the other case study.
Michael
Sure, so a non-monetization study, right? If we're thinking about monetization, as the externalized realized value of data, we can look at, like, let's say, I'm good with my revenue streams, my problems are not my revenue streams, how can I use my data to improve the employee experience and prevent attrition?
Vinnie
Everyone is suffering from The Great Resignation, right? So did this happen pre or after?
Michael
Pre.
Vinnie
They set themselves up pretty well? Right?
Michael
Yeah, they had a pretty technologically advanced business model. This case specifically applies to a ground logistics company. So, any of the large trucks, vans, carts, anything that moves parcels from one place to another, this company hired the owners of the part of those vehicles to move their shipments from A to B. What the management company actually was facing was a lot of those contractors, a lot of those employees were burning themselves out and ultimately quit, which resulted in things not being shipped. So, a logistics nightmare as part of this great resignation and these folks are the heartbeat of the supply chain. What they tried to do was answer, what's our value proposition to these contractors and how do we get these employees to stay with us? What can we do to prevent them from burning themselves out? Looking at all the data points of a route, my relationship to those routes as a driver, and trying to find patterns. Most of the attrition that's occurred in the last 2,3,5 years has been preceded by a pattern of less frequency of trips, the overall earnings from my same trips going down, the safety of the route I'm driving, decreasing, etc. Among hundreds of other data points, obviously, right? Pretty sophisticated kind of advanced analytic predictive model going on here. You get the idea of what they're trying to look out for in terms of this pattern is to behavior and to the point of preventing it. Having HR reps reach out and offering solutions to say, “hey, we've noticed this pattern. It's degrading a little bit. How can we help?”
Vinnie
Yeah, I think of two things there, well more than that. One is if we go back 10 years’ time before this sort of maturity, what we're talking about is data science, right? A traditional way to do that would be to ask people what the five factors are, or the 10 factors are when you say hundreds of factors. We're now asking the software itself to come up with pattern matching and telling us and it's a much more powerful thing, because it's including information that would have been gaps before. The other thing that strikes me is that we're not reporting necessarily, you are. These are the reasons but you're going that extra step in the maturity model of predicting behavior. That really is the top of the maturity curve or towards the top of the maturity curve when you can, when you're looking forward, not backwards at your data
Michael
Yes, absolutely right. If you think of the data productization funnel, right at the top is the net new product for that new customer and the predictive aspect of that is predicting customer behavior, predicting product needs. And predicting the data points needed to answer that. That to me is the future of data productization right there. Leveraging algorithmic expertise and data science and this advanced analytic capacity to, remove the retro thinking out and enable your human analysts and engineers to focus on forward-thinking problems and let the system figure itself out and give you a report back of, hey, here's what's happening.
Vinnie
So, let's use this case study to generalize it. And we'll use it to go backwards and say, what needs to be in place? What did they do right? That got you here. By the way, we said this wasn't a monetization story. But it's not an external monetization story. There's a lot of money saving happening.
Michael
Yeah, just to tie a bow on the story, right? The value there was employee satisfaction. They want to be the best place to work. Obviously, retention saves a ton of money but that wasn't the ultimate drive. It was really making sure that their people were okay, and can they prevent any degrading patterns that might impact someone's livelihood, from happening.
Vinnie
Right. That's great. So, let's work backwards. There's employee data, there's job data. I'm imagining there are several different sources of data in this model. There has to be enough data, the right data, and trusted data. Was all that in place? We don’t have to get specific with this client. Let's just go generally, generally, when you come into places, is it a mix of maturity, where it may be? I can imagine some things have been acquired. Some things are legacy, some things are new. So, I'd imagine it's a hodgepodge of maturity.
Michael
I was going to say hodgepodge. Yeah, exactly. Most of what I see is there are pockets of maturity, there might be one group that's doing everything to the tee and now they're looking at academic excellence on can we do hourly deployments instead of daily, while meanwhile, there's a group back here still working off Google Sheets and handing physical pieces of paper down the hall, right under the same umbrella. So, it is a hodgepodge. Is that enough to get started data productization? Yes.
Vinnie
Okay. So that's, I think that's surprising to a lot of people. I think that the analysis paralysis, of our data, is not perfect. look by the way, no, one's data is going to be perfect. When people say, when is the job done of getting good clean data? It's never done. There are always new systems, always new stuff.
Michael
Yeah, and that's, proliferated in any advanced analytics space because you want your model to be 1,000% more effective, right? Sometimes you kind of lose the forest for the tree there a little bit. In terms of data productization, all you need is a trusted data source, you need trusted data source, and you need a line of sight into a piece of your value proposition. The relationship between that data and the value proposition. You don't need enterprise data governance at scale, it helps to get up that maturity level of data productization, to bring net new product, net new customer, you will need the enterprise data governance in a certain spot, you need your entire data ecosystem, data fabric to be trusted and you need to be able to bring in third-party data as needed in a similarly trusted way. There's your hyper mature level. To get started, you need some trusted data somewhere.
Vinnie
Can you define data fabric for me?
Michael
Data fabric, talking about buzzwords. It’s sort of the same thing as a data mash. It's anywhere that your organization has domain over data. It could be your IoT device producing a signal to your, ERP employee system, producing employee data, it can be your transactional systems.
Vinnie
You're saying it's the macro domain of all data, including bad formats, like Excel spreadsheets. So, it is just not the well-architected domain, it's well-architected and poorly architected domains. Not that Excel is poorly architected.
Michael
There are small companies that can work off Excel. We're not saying we need a full-blown cloud solution for every company everywhere. That's the beauty of data productization. It's not necessary. It's a best fit solution to drive net new value from your existing data assets. So, if you are a social media-based business, your product is insightful tweets, you can do your back-end magic and have all your data living in a Google Sheet and publish it to Twitter or publish it to paid Twitter to generate your revenue, right? That's perfectly fine. And especially if it's one and a half people or one person and an intern working on that, that's pretty valid.
Vinnie
Thank you for making that distinction. I think going back to the beginning of the buzzwords and the buzz phrases, you can hear these things, and a lot of the white papers you'll read or position papers by product companies, it sounds big. It sounds very involved, and it can be, it can be very big and very involved, but it doesn't mean there isn't work that can be done immediately and value that can be gotten immediately. Let's finish with a couple thoughts on platform and how to get started. We've had podcasts about this before, too. A lot of trend thoughts on this, where moving to the cloud, if you avoid a lift and shift operation, also means modernizing platforms and processes. I feel like it's Google Cloud or Azure or AWS, there are mature areas now for this type of data science and analytics work. Two questions. One, is this work primarily done in the cloud? Are there still a lot of on-prem people? And I guess there has to be for some of this legacy stuff. And too, is there a preference between the cloud vendors? Or are they all pretty much offering something that's good? And if you're on a particular cloud vendor, you're probably okay.
Michael
Start with the first question. You can't run a modern data product off of legacy systems. So, if you have an Excel spreadsheet that's extremely valuable, how am I going to pay you for that information? How are you going to deliver that information to me? That's a question. You do need cloud capability to a point of, you know, API driven stuff is pretty ubiquitous at this point. Up to that point where you can deliver in a modern way, in a modern facility. That's the low bar, right? There is a space for hard artifacts in the data productization landscape where you're adding value, it's a piece of the puzzle that adds value or creates value from your data assets. Ultimately, yeah, you do need a pretty modern delivery mechanism for that. The second question is, are any of the big cloud providers…
Vinnie
uniquely distinguishing themselves or does it come down to use case?
Michael
For data productization and the basic level, they're all great, they all do the job. Interestingly, when you get to the point of third-party data onboarding, and what I've experienced, is if you have one cloud provider over the other if you're negotiating with a strategic third-party data source, and they are the other cloud provider, or they don't think highly of the cloud provider, your team has chosen, that's a bit of a bargaining chip at the table, right? There's some nuance there. From an objective technical perspective, all three clouds, all major cloud providers, AWS, Azure GCP, all have tools to do the job.
Vinnie
So, it does come down to, there's other reasons to choose cloud vendors. That shouldn't be the determining factor, correct?
Michael
Yes.
Vinnie
Great. So, let's wrap up. First, thank you for coming. I want to hit key things to take away how to get what can people do over the next, four to six weeks to make progress here? I'll hit a couple from a top-down perspective. Maybe you can hit a couple from the bottom-up? Do you have a CDL? Is there a person who's representing data in your organization? Do you understand or have thoughts on how that data can tie to a corporate vision? Is it something that can be monetized? Is it something that adds value to a process? And I've said this before, but having a seat at the table as an equal person in that digital transformation, I think is a good top-down, way to start. Give me some good sort of grassroots tiger team bottom-up kind of stuff that people can do if they are just starting off on this.
Michael
Again, identify your trusted data. First and foremost, from there, I think the flip side of the coin, identify where you have known gaps in your data, where do I not trust the data? Why do I not trust it?
Vinnie
So, you're talking basic data engineering?
Michael
Basic data governance, basic data fundamentals. Organizationally, that's where I would start if the idea was let's see if we can take action or find some new action to take against our known data. Obviously, having line of sight into corporate values and vision. That's top-down and bottom up.
Vinnie
Well, I think in the middle where they meet is a quick win.
Michael
Kind of hitting singles. Hitting singles that are going for the homerun, that's a scale you’re thinking back to a simple use case first, and build from there.
Vinnie
Yeah, I mean, this is true with a lot of other technologies. When you say simple use case, I sort of have that four-quadrant view of like, what has a lot of value but lower complexity. That can be something that has super high value to a small group of people or some value to a lot of people, right? Yeah, am I going to go narrow and deep or shallow and broad? You know, if you're in that unique situation where you can do something super valuable for a lot of people and its low complexity then that's the best but otherwise, you got to figure you know where you want to target maybe you have a couple of different singles. We have a single here, a single there, so you're hitting a couple different audiences. Yes, that goes back to the audience thing that we talked about earlier. Well again, thank you for coming. Very insightful, enjoyed having you on the podcast.
Michael
Thanks for having me.
The entire contents in designing this podcast are the property of CapTech or used by CapTech with permission and are protected under U.S. and International copyright and trademark laws. Users of this podcast may save and use information contained in it only for personal or other non-commercial educational purposes. No other uses of this podcast may be made without CapTech’ s prior written permission. CapTech makes no warranty, guarantee, or representation as to the accuracy or sufficiency of the information featured in this podcast. The information opinions and recommendations presented in it are for general information only. And any reliance on the information provided in it is done at your own risk. CapTech. makes no warranty that this podcast or the server that makes it available is free of viruses, worms, or other elements or codes that manifest contaminating or destructive properties. CapTech expressly disclaims any and all liability or responsibility for any direct, indirect, incidental, or any other damages arising out of any use of, or reference to, reliance on, or inability to use this podcast or the information presented in it.