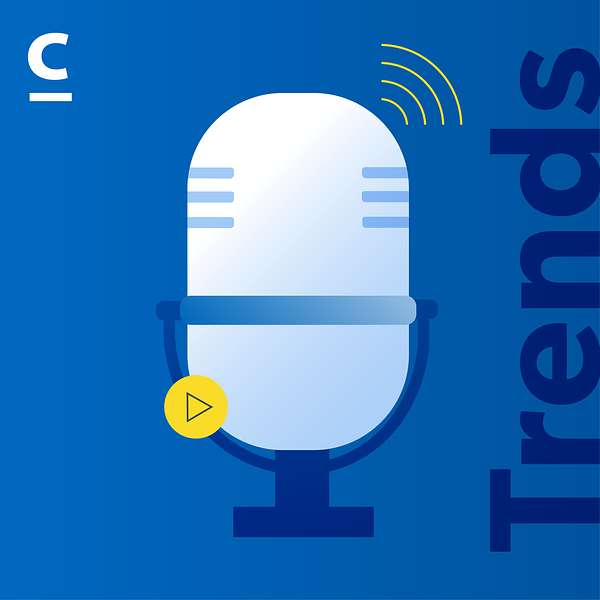
CapTech Trends
CapTech Trends features thought leaders and subject matter experts discussing emerging technology, design, and project methodology. Our goal is to unite diverse skills and perspectives to show how data, systems, and ingenuity can transform and enable organizations to advance what’s possible in a changing world.
CapTech Trends
Kickstarting Advanced Analytics
Are you getting value from the data that you have? Listen in as Vinnie chats with Andrew Novokhatny about moving from traditional to advanced analytics. Andrew is a data and analytics expert and healthcare analytics professor at UNC Chapel Hill.
Tune in as we discuss:
- Pivoting into forecasting and making predictions using your data
- Don't forget the change management part of the equation
- How high-performing companies are using their data
- Healthcare use cases in the fraud, waste, and abuse domain
Vinnie
Welcome back to the CapTech podcast. Today we're going to be talking about kickstarting advanced analytics, and with me, I have Andrew Novokhatny. In addition to being a senior manager in our data and analytics practice at our Richmond office, he's an adjunct professor teaching healthcare analytics at UNC Chapel Hill. Welcome, Andrew.
Andrew
Thank you. Glad to be here.
Vinnie
Yeah. So what we're going to get into today, talking about advanced analytics, given some case studies, how to get started, et. But what I wanted to start with was just basic regular analytics so we can contrast between what normal analytics are and what advanced analytics are. So to kick that off, short of a definition of what analytics are, walk me through what that looks like.
Andrew
Sure. So yeah, we define traditional analytics as more descriptive in nature. You think of yourself looking at an Excel sheet with lots of columns and rows, obviously representing a variety of different things. For traditional analytics, we would take that Excel sheet, and we would maybe just do some basic statistics on it. We'd find means, medians, averages, that kind of thing. And as we move into a more advanced state, you would then take that same Excel sheet, and you'd try to forecast and maybe add in some more complexity in predictions and trying to draw conclusions from that data.
Vinnie
Gotcha. So instead of being declarative against the data, you're using it to make predictions.
Andrew
Right. So yeah, if you want to think about it in a more definitive way, descriptive analytics looks backwards, it's backward facing, while advanced analytics typically faces forward and is used to predict things that are going to occur generally in the future.
Vinnie
So my first thought is, well, that means traditional analytics are more accurate because if you're making predictions, you're not going to be a hundred percent right. So does that change, now there's power to that, but does that change, I guess, where and how you apply these technologies?
Andrew
Yeah, that's a really great insight. The real nuance there is finding out why a given set of data is giving you the conclusion that you've just identified. Oftentimes for any advanced analytics application that we build out, we really try to understand what is causing a given metric to look the way it is. And based off of that reasoning, we actually then tweak models depending on exactly what the descriptive statistics are telling us because it helps guide our actual decision-making going forward.
Vinnie
So in both the companies where you've done this kind of work and just general industry knowledge and teaching, are people using off the shelf models and algorithms, or is there still some innovation happening within these organizations? Basically, is it about the data you collect and applying the models that exist and the algorithms that exist? Or is there still innovation happening within these large companies?
Andrew
Yeah, no, there's definitely still innovation with the advent of really all the cloud services offering their own marketplaces now. I'll use AWS, or Amazon Web Services, as an example. So they provide algorithms off the shelf for specific use cases, whether that be healthcare, financial domains, etc. They wrap in the data intake into those off the shelf packages, but they also have a pre-trained or ready to go algorithm that's specific for that specific domain to use for those kind of use cases.
Vinnie
We had a podcast, gosh, that must have been a year or so ago about asking the question, is there a risk if everyone's using the same models that you're basically predetermining the kind of answers and predictions you're going to get, and then you're lacking some competitive advantage because we're all using the same thing. Am I hitting on something there?
Andrew
Yeah, I mean, there's certainly something there. You'll find that more in the field of something like computer vision. So whenever you have anything that is really trying to identify what a type of image is as a prediction, that generally involves you having to collect massive sums of data, whether it be off the internet or elsewhere. And really companies like Google and Microsoft will simply sell you pre-trained data sets so that you can use their information to generate those outcomes.
In the situations that we really see for our clients and some of my academic work, typically corporations and organizations have their own data, and they'll use that, their own confidential proprietary information, to build their own models. So really, you'll still see innovation and a competitive advantage in the market.
Vinnie
So the innovation and the differentiation comes from your ability to collect the right types of data with all your five Vs of data, enough of it, enough variety, the right kind, I mean, it seems like that's what you're saying.
Andrew
Yeah, yeah, pretty much. It's really obviously specific to the use case that you're looking for, but really the sausage is made, so to say, in a lot of these industry applications and the modeling teams that build them is how they collect the data and what they decide to use for training. And to add a little clarity, so training is the practice of exposing the data that you've collected for a specific use case or solution and exposing it to an algorithm which then identifies the patterns and makes predictions off of those.
Vinnie
How do you know if you have the right data, good data?
Andrew
Lots of iterations, essentially throwing, I always use this analogy of throwing spaghetti at the wall, and eventually one of your noodles will stick, and that noodle being a model or some sort of proof of concept, prototype that you've developed. It's really no different in any organization you go to. You've really got to go through a lot of different iterations to see what works and what doesn't.
Vinnie
That's interesting because, again, that makes me think, wow, change management's going to be tough on this because if you decide, as a company, to move from basic analytics to advanced analytics, you're not going to get it right the first try. You're going to have to try and fail and learn, test and learn, test and learn, test and learn, and then have something that's pretty cool. So the organization has to understand that that's part of the methodology.
Andrew
Oh yeah. Organizational buy-in is one of the biggest elements that we try to push whenever we end up completing work like this with some of our clients. Really, it's getting a whole team to understand the purpose of a given application and understand the use case and the usage for it. So really getting buy-in from not only the folks with the purse strings who are actually providing money for a given initiative, but really the modelers, the data collection, individuals who are collecting the data and anyone really involved in the entire pipeline or process.
Vinnie
When I do strategy work, one of the things I see as a common thread, speaking to this change management is a lot of organizations, especially on the business side, they don't know what's possible with advanced analytics. They don't know what types of questions they can and can't ask, so that makes the adoption more difficult as well. Because if we don't really know how we can apply this technology, then we can't assign a value to it. So when we talk to clients, are you seeing that same thing, first of all? I want to validate my experience on that. And second, how can companies become more aware of what they can be using these tools for?
Andrew
Yeah. Most companies in general have their broader domains and just a few generic use cases, I'd like to say, within each of those domains that they know AI is can be used for. Really where we see the differentiation between the organizations that are what we'd call high performers, really understand the holistic aspect of things. It's understanding all of the different team members that need to get involved. It's not only siloing the modeling task to the modelers, but incorporating the end users in the process, incorporating from a change management perspective, making sure the managers, just the people managers, are aware of the bandwidth required in order to work through collecting data, training a model, and then even deploying it later on. Yeah, and I think I'll dive into a little bit more detail, specifically the nuances that we see between the low performers and the high performers in the space.
Vinnie
Let's do that. Go ahead.
Andrew
Yeah, sure. So I mean, there's a lot to really flesh out here. So if we think about it as a process, from the data collection standpoint, if you're an organization that sits lower on what we refer to as the information maturity curve, you may only be collecting information in Excel sheets or spreadsheets and don't really have an organized central repository of data. I think you've had previous episodes where you've had folks come in and discuss data marts or data warehouses and stuff like that.
Vinnie
Sure.
Andrew
Those are really, really awesome tools that provide your modelers and your teams with an absolutely expansive set of information to work off of and try to generate new ideas. So what we see is the high performers really understand the complexity required in standing up those resources and then using them for different applications amongst different lines of business even. The low performers typically only, and I hate using the word low performers in a way, it's really, what's a good way to put this?
Vinnie
Low leveraging.
Andrew
Yeah, yeah. They're simply not, exactly, they're not leveraging the data that they already have in a structured manner. So yeah, it comes down to infrastructure often.
Vinnie
Yeah, I've got an image in my brain, like a horizontal stacked architectural diagram where getting that data into a trusted technology stack is the foundation, and then different things can sit on top of that. So advanced analytics can sit on top of that. But then I'm assuming too that generative AI can also sit on top of that. So I guess my question to you is, we look at AI and generative AI and advanced analytics, and they're different things, but every time I talk to an expert about it, it seems like a lot of the foundational elements are the same. Is that true?
Andrew
Yeah, at a high level it's a true statement. I think at this point in time in the field, there's really all of these different sub-domains within artificial intelligence and machine learning really have their own set of expertise and experts that are aligned with them. Generative AI, as that has become popularized most recently with ChatGPT in the work that the open AI group has done, has really brought a lot of this AI to the forefront and to the public space, and there are a lot of overlaps and similarities, but behind what we do in advanced analytics and what you can do with generative AI.
But in general, the premise is the same. You take a lot of information. You take a lot of data. In the case of ChatGPT, you scrape the internet, and you find almost every different example that you can of various documents, code examples, recipes, email templates, and you condense them as different parameters for your model that then will generate outputs that are based on things it's seen previously. The way we leverage data for our training and for our use cases in advanced analytics are kind of similar. The algorithms are different, and the way in which the actual outputs are generated are different. The premise is the same. Take data, expose it to an algorithm, provide some sort of forecast or prediction.
Vinnie
Right. What are some typical use cases that you see, so we can take this a little bit closer to what people can experience?
Andrew
Yeah. So for the sake of brevity, I'll just give a handful because there's use cases all over the place in regards to AI or machine learning here. I'll start with describing use case that can be leveraged across the financial domain and can also exist really in retail as well. And that's customer segmentation is a really easy thing for most folks to grasp, even if you're not necessarily data savvy or statistics savvy. But in general, it's taking large corpus of data and identifying patterns within that data set to potentially add in classifications for your, let's say, customer profiles. Is this a customer who happens to be a very loyal individual who constantly comes back and purchases products for us? Is this somebody who maybe has just arrived to our website for the first time? If companies or organizations can identify those individuals quicker and faster, they're able to essentially send those predictions down the pipe in order to engage those folks in a different way.
Vinnie
Can that be done real time?
Andrew
Oh, yeah. It can definitely-
Vinnie
So I'm calling into a CRM, or I'm in a chat bot or whatever else, and you know I'm a loyal customer. You know I'm a high spender. You know I have something in CRM not working. It's like, get this person to a real person.
Andrew
Correct. Most websites that you visit on a regular basis to purchase anything from typically have something like that running under the hood where they're taking your click data, they're sending it to a backend system, running it through an algorithm and returning a result or a prediction of you as your customer segmentation profile within the matter of seconds.
Vinnie
Gotcha. Great example. Do you have another one for me?
Andrew
Yeah. So my subspecialty is really within healthcare. It's in the healthcare analytics domain. And really, the implications of being able to take a large section or large collection of data and be able to draw conclusions from it as a powerful tool. Public health agencies across the world, and even health insurers, are using this in the form of health surveillance to identify essentially patients at risk, which has benefits not only for a public health agency such as a state department of health, but really for the insurers as well. If the payers themselves can identify at-risk patients to provide an intervention before that patient incurs more costs that become prohibitively expensive for a given set of claims that they might have to reimburse on, they can send out care managers early on to identify what the problem is. Do they need help with medications? Do they need help with other things related to their health? So really the use cases are endless.
Vinnie
Yeah, there was one I read about a while ago, and I might have mentioned this on the earlier podcast as well, but it related to wearable technologies like a watch, for instance. And senior citizens who fall and break their hips exhibit very small changes in how they walk days leading up to that event, how long it takes to go up and downstairs, if you're favoring a side or another side or minute things. But using that data, they can then predict you need to come in for some preventative care because you're only days away from having a fall. But I bring that up as a way to ask the next question, which is, all the HIPAA concerns, all the privacy concerns. Can we share this information generally and use it to make predictions, or are we not allowed to grab it generically?
Andrew
Yeah, that's a fun question. So where this gets complicated is really what the FDA defines as a medical device. If you are running an algorithm under the hood of some sort of wearable product that is formally considered a medical device by the FDA, you fall under a very set of specific rules that you cannot break. It is a very different use case than if you're simply providing some sort of potential evaluation of someone's gait without recommending a treatment or a prognosis, then you fall into a different bucket. But yeah, your point stands, and I think especially with what we're seeing with GDPR and the various different data privacy laws that are being put in effect in Europe and the United States as well. We'll probably see more restraints coming within that system probably in short order.
Vinnie
Can you get by those constraints simply by having an opt-in? Are people allowed to do it if people agree to it?
Andrew
More than likely, assuming you've got deanonymized, or sorry, anonymized data sets, and that folks can't really trace back to exactly what's going on. But the real key distinction is that as long as the FDA continues its set of rules that it has for medical devices, you're really limited in exactly what you can recommend and the types of things that you can do with that data from a prediction and a forecasting standpoint.
Vinnie
Gotcha. The other use case I think of a lot and see a lot, and it spans almost every industry or probably every industry, is fraud, waste, and abuse. So help me understand how this technology helps in that domain.
Andrew
So fraud, waste and abuse is a really fascinating domain, especially for advanced analytics use cases. This is something we see not only in the financial space, but also health insurers have entire corporate divisions specifically dedicated to identifying these revenue streams of returning money back to the coffers, so to say. And so really this is almost the perfect use case to describe the transition that the industry's gone through from the traditional descriptive analytics that we described earlier into the more advanced space in leveraging these new tools. So when you think about any generic fraud, waste and abuse recovery pipeline, whether it be at a bank or health insurer, typically what occurs is claims or financial records are flagged for review. That flagging could be done off of just basic rules. So did somebody send money to a country that they weren't supposed to? If you think of almost just traditional database queries like, hey, we're looking for this amount of money that was sent this many times, and it's just a basic rule that somebody broke. If you get flagged for that, you eventually get sent down the pipe to an investigator, which is a real person, usually somebody with a law enforcement background that then is able to scan all of your information, identify whether or not there's actually a case to build, and they'll eventually send it off to litigation if it's worthwhile. What we're seeing now in the industry is they're beginning to augment the processes and the actual workflows that they have now to incorporate advanced analytics to help them flag these claims and financial records earlier on. So if taking the example of health insurance, if you can flag a claim before it's paid out, before that check is mailed out of the door, you save an inordinate amount of money on recovery, on the investigation side, on litigation because you haven't paid it yet. You're able to identify if it's a fraudulent payment way ahead. And so you're essentially just sending all this information down to the end users, as we call them, the fraud investigators, and you still allow them to make their own decisions with it.
Vinnie
That goes back to what we said earlier in this podcast, whereas as this is being predictive and that changes the workflow. See, that was a great example of how the workflow changes as a result. You're not just automating everything and shutting it down. You're getting the human involved with the right data at the right time. That reminds me of another example I read about, and I'm curious if this is similar to fraud, waste and abuse technically, and that is having artificial intelligence review x-ray and CAT scan type information for tumors or other medical concerns, and then doing a lot of that bulk work and then handing it off to a medical professional to actually do a review. I'm sure you're familiar with that. Is that similar technology, similar workflows here?
Andrew
Gets a little trickier, but yeah, generally the same premise is applied here. So you have, obviously, a process where a computer is making a decision at some point as to whether or not a given disease may or may not exist, and you send that off to a radiologist. I think I've read articles recently where apparently radiologists may become obsolete in the near future or something to that extent. And really, what we're finding is that's absolutely not the case. It's simply another tool in their toolbox to help them increase the amount of actual images that they can get through, the amount of studies is what they refer to them, while still continuing to essentially utilize the same workflow that they had previously and providing a diagnosis at the end of the pipeline. So by no means are radiologists disappearing. They're simply leveraging new tools to help them increase their thorough put and allow for the diagnoses to keep flowing.
Vinnie
Yeah. So I'm thinking for the audience listening to this, these are good examples, and it's a good challenge for you to think about your own organization and say, okay, how does this apply to what we do? So stepping into that, to round out this conversation, people may be listening to this, they may be interested in the use cases, but still not know exactly how to affect change in the organization or what's possible within the organization. What are the first three things you do if you're coming off this podcast, and you're interested, and you want to do more?
Andrew
Yeah. So really I think the first step would be identify where exactly your organization or group sits in that information curve. So are you just doing really basic data collection and existing in the world of spreadsheets? Or really, are you a little bit more advanced, and maybe you're actually collecting things in a database. Maybe you already have a data warehouse set up. The next question you should ask yourself is, are we actually getting value out of our information and the data that we have? So really identifying whether you have a strong foundation of the information and data in general is a really great first step. That'll essentially help identify whether or not you need to bring in more resources to help build that out further, or if you can move on to the next step, which is generally, do you have the skills necessary to use that data? So what that essentially entails is finding out if you have analysts or if you have engineers and modelers that are able to extract that information and then make predictions or deploy them in some manner.
Vinnie
Are data scientists part of this as well?
Andrew
Data scientists are part of this as well. This has become an interesting topic in and of itself in the titling of all of these different individuals within the world of advanced analytics. You'll routinely see titles such as data engineer, data scientist, and now more recently, machine learning engineer, who all have very different scopes of practice, so to say, but generally work within the same units and teams. So understanding, and maybe even working with a trusted partner to help you identify what needs you have within your organization is certainly not a bad idea.
Vinnie
So you have to have the data. You have to have the expertise. What else do you need?
Andrew
You need to find out what you're going to do with it.
Vinnie
I think that's the hard question for most people. So let's get into that before we wrap up. How do you know where do you go? Who do you talk to to find out what you can be doing? Is it basically comparative and competitive analysis to go out and see what other people are doing? If so, you're seeking parody, which isn't horrible. It's probably a good first step. But how do you know what the possibilities are?
Andrew
Yeah, that's a fun question and something that we've actually, I think the whole industry has struggled with because when you really think about it within the corporate world, most low-hanging fruit has been picked off. Most organizations are climbing the tree, so to say, and really digging and looking for things that they can identify to optimize and incorporate a little bit of leaner practices that may might have advanced analytics involved.
Vinnie
And I realize that question's rather rhetorical, and I'm asking for the silver bullet.
Andrew
Yeah, yeah.
Vinnie
But I don't think there's an easy answer to that question. I think there's a lifetime of work around that question.
Andrew
And we've actually had really great experiences with, we've been referring to them as brainstorming workshops, where we bring in not only the end users. So let's take for example, the example we had earlier, fraud, waste, and abuse. We blocked off half of a day. We brought the whole team in, and the team included the fraud investigators themselves, some of the folks that ran the database or the data warehouse, and we brought the modelers in as well. And we had a semi-structured format in a way where we simply picked off those low-hanging fruits for use cases and potential solutions. And then we just talked the rest of the time and wanted to understand exactly where else they could fit in. Being able to have a modeler in the same room as somebody who knows nothing about statistics but is an individual who actually drives the work every day, day in and day out, is a really unique experience because you get to see two people really getting to a conclusion of how to better use technology in their day-to-day lives.
Vinnie
I can see that if you're going from basic analytics or advanced analytics. Do you need more roles, or do you need additional skillsets for the people that you have?
Andrew
Yeah, it's not even so much as hiring extra people or getting extra roles. It's really just getting, going back to this idea of organizational buy-in. When everybody on a team is aware of why something is getting built, they really understand not only the reasoning for it, but they understand how they can impact it, and they can modify the inputs in terms of whether it be data or how you're solutioning some of these things. So we found that in general, it's been a really positive process. And once everyone feels engaged, they all respond really well to the whole situation. And you get good solutions out of it.
Vinnie
So last question for you. When I'm trying something new, back from my developer days, I like fast iterations. I like proof of concept. I want to take the most difficult things and get them done upfront and showcase a win quickly. When I talk to people in the data space, we always hit on this foundation of lots and meaningful data. That seems like a heavy burden if you don't have that to even get in and start playing around. So if you are lower on the maturity model here, is there a pathway to say, hey, let's carve out two to three months and show some value? Or is it, nope, we have to do a 16-month project and get the data right? How can we force someone who's on the bottom part of that curve, is there fast iteration type proof of concept, get people inspired, things you can do?
Andrew
Yeah. So we actually apply the same methodologies and processes to both the high performers and the folks that are kind of lower on that information maturity model. So prototyping as a field, in and of itself, or specifically as a topic within the concept of modeling and deploying data science applications is something that you need to do quick and fast. You need to be not afraid of failure, and you need to be able to also understand when to pull the plug on a given idea. It's something that sounds scary when you start working through it, but once you start realizing that, you'll generally have more ideas than you have time or data to do something with. So you might as well, as I kind of mentioned earlier, throw spaghetti at the wall and essentially find out what sticks.
Vinnie
Right. And then you're also probably learning more about what's possible, and more spaghetti is more likely to stick over time.
Andrew
Exactly. Yeah.
Vinnie
Gotcha.
Andrew
And that concept of rapid iteration is really, at its core, something that both anybody at the lower end of that maturity model can use, and also what our high performers are using as well.
Vinnie
Gotcha. Well, thanks so much for joining me. I'd like to have you back at some point and talk more about the operational side of this, get into the weeds on MLOps and things like that. For the audience, thanks again for tuning in, and we'll be back soon.
Andrew
Thank you.