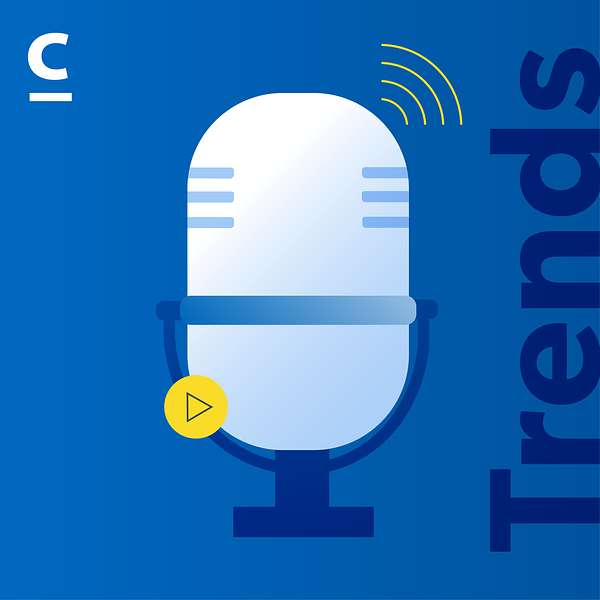
CapTech Trends
CapTech Trends features thought leaders and subject matter experts discussing emerging technology, design, and project methodology. Our goal is to unite diverse skills and perspectives to show how data, systems, and ingenuity can transform and enable organizations to advance what’s possible in a changing world.
CapTech Trends
Navigating the AI Evolution: A Discussion with Dominion Energy, Virginia Commonwealth University, and CapTech
In this special edition episode of CapTech Trends, guest co-hosts and CapTech AI leaders Brian Bischoff and Jason Snook are joined by Ajit Mandgi, the Director of Analytics at Dominion Energy, and Paul Brooks, Professor and Chair in the Department of Information Systems at VCU School of Business.
Tune in to learn how AI is impacting various industries and gain helpful insights to navigate the future as this technology continues to evolve. In this panel discussion we cover:
- How ChatGPT has impacted higher education and how educators have quickly adapted their teaching strategies.
- The transformational impact generative AI has had on the energy and utilities industry.
- The responsibility of leaders to help organizations embrace and navigate AI - and keep up the momentum.
- What the AI transformation will look like in the next few years.
Brian
Hello, and welcome to CapTech Trends. First of all, I want to introduce myself, I'm Brian Bischoff, and Vinnie Schoenfelder is not here today, but don't worry, he will be back for future podcasts. And today, we are talking about continuing our series on AI and artificial intelligence, and we have brought in a client of ours, Ajit Mandgi, and we also have Paul Brooks from VCU. And so, great to have different perspectives as we embrace this new era around AI that we're in today. So maybe I'll start off with just a quick introductions, also have Jason Snook with CapTech with us, as well, so Jason is going to join us again from the AI land and talk through us with AI. So maybe I'll start with Ajit. Ajit, why don't you do a quick introduction on yourself?
Ajit
Sure, I'm Ajit Mandgi, I'm the director for analytics at Dominion Energy. I've been involved in BI and data analytics from the last 20 years, and we have been developing some exciting AI solutions. Looking forward to the discussion today.
Paul
I'm Paul Brooks, I'm a professor and chair in the Department of Information Systems at VCU School of Business. I am also the founding co-director of Center for Analytics and Emerging Technologies, and my background and my research has been about using optimization to develop machine learning methods, mostly, and more recently, we're also using conjecturing methods to discover patterns and data.
Jason
Fascinating. Yeah, we were excited about this conversation, because we've had a bunch of AI podcasts, and we've talked about what we're doing as a company, and we've talked about what we're hearing from other clients and organizations, but it was all secondhand, and so, we thought, well, we want to bring more voices in, we want to hear kind of firsthand, what folks are doing. So we were excited to have you guys come today.
Ajit
Thank you.
Brian
So in your intros, each of you have indicated you've been in this advanced analytics space for quite a long time, and this year, it's been brought to the forefront, the whole GenAI movement, large language models and everything, has really had everybody refocus their attention on a lot of things that are happening in this AI space. So I'm curious what you're seeing right now, just given the space that you've been in, the history you have, what are you seeing right now in each of your domains, related to AI, and how maybe it's changed in the last year?
Ajit
Well, at least at Dominion, there's a lot more interest, because this has become more publicized, and everything else, with ChatGPT, and all this AI, has come to the forefront of all discussions. So that is always welcome for us because we can now use that to better leverage the technology to help with managing our assets in the field, and everything else, in a better way. So I think it's all for the good.
Paul
We have a theme this year of disruption, and we are totally disrupted by it in higher ed. It totally changes the classroom experience. I'm constantly running up and down the halls to faculty members, we're trading notes on what products are available, what products are no longer available, what they're called now, what they were called last Tuesday. I walk in the classroom, and I say to the students, okay, we're going to solve this problem, try to get the AI to solve it. And it turns out, they're not that good at using it yet, so they need some coaching. So then I say, okay, we'll solve it from scratch, and then we go back, and then we talk about how to coach the AI to get it right. Because then, when we show the students how to do it, they're like, well, I'm done with that AI thing, and gets it wrong. It's like, no, no, no, it's still a good starting point for you.
Jason
Interesting, yeah, and I'm sure buy-in ... I'm hearing that a little bit, buy-in's a little asymmetrical. Everybody in this room is very excited about AI, but I mean, are there pockets where people aren't as excited? What are the concerns that people are giving you if they're not moving as fast as the people in this room are?
Ajit
One of the things that is challenging with AI is to be able to explain how the solution is coming up with its ... Especially when it comes to predictive analytics. So they're all used to the descriptive analytics of what happened, and showing all that, to be able to use that and start predicting, that is something new. And they always say, so what did it weigh heavily on? Why did it decide on giving more importance to this particular factor? And that explainability is something which is always going to be difficult to solve when they talk about it. We do show what criteria might be influencing it, but when it is a synergy of all of those things, it's difficult to say what the weightages are. Some algorithms, we do know, some tools, they do give us, but the explainability is a challenge.
Paul
Yeah, I want to add to that. I mentioned I use optimization to develop machine learning methods, and that's almost going out of vogue. But with optimization, we write down what we want to do, and then we build a model to do that, but with generative AI, it's kind of an artifact, deep learning is an engineering artifact that we can't really explain very well.
Ajit
Yeah, and when it comes to this foundational AI, as you call it, we don't really train it for any one use case, like this ChatGPT, and all that. But [inaudible 00:05:25] days, we used to look at the past data for a certain use case, and we used to train the models, and it used to predict on those, but with this foundational models, it is like it knows everything, supposedly. And then, you have some gotcha moments where it might not be responding correctly, because probably, there is not enough data it has been trained on, or what, it's difficult to analyze there.
Jason
It's interesting, though, I think there's a lot of little things, and we'll probably get into this, that you can do with AI quickly, but a lot of the big targets, the big things that we could do with AI are blocked by explainability right now. You're not going to use it for admissions right now, you can't really use it for-
Paul
Oh, yeah?
Jason
... demand forecasting. You've got to be a lot more careful until we can explain it. I think there's a lot of money and interest and effort in cracking that. So the next couple of years will be interesting, because we can't just keep shrugging our shoulders, right?
Ajit
Yeah, how do you explain to the regulators why a certain forecast is being picked? I mean, that's very interesting, I mean, what you bring [inaudible 00:06:28], but what we have to do is use it as more a supporting mechanism to what is being developed through rules and understanding of the fundamentals of the business there, yeah.
Brian
And Ajit, what you mentioned, and Paul, you've hit on this, also, is that you used to build these models and train them on large amounts of data, or maybe not necessarily large amounts of data, but test the results of that over and over again until you get the prediction level that you expected, that you can entrust in the majority of the scenario that represent the outcome you're looking for. Now we're talking about these large language models and things that are happening almost in real-time. You're putting information into these ChatGPT, the generative AI types of models, and they're bringing back information instantaneously, based off of whatever information you're providing at a time. And that explainability, to be able to explain what's happening in the moment right there, is extremely difficult to do, right?
Ajit
True, that's very daunting, especially if you are the data scientist. But we have definitely seen a lot of benefits from AI, and it has cut down on a lot of this extra manual work which was being done. People used to hold data, get in all the data, try to do their analysis, and multiple people used to come together, and their analysis did not match because the weightages were different, whereas these things are very good, especially when we start analyzing it from the past data. I love the concept of how you have a holdout data in machine learning and AI, that is like, they take last year data out of the training, and they ask you to predict the last years, because the results are known. And to then see, how accurate was it, they cannot do it through precision and recall, the two parameters on how good it is.
And all these concepts are very much usable in other areas, too. Trying to understand, how good is our guess? I mean, even if it's a guess. But in AI, there is surely the science behind that on how it's done. And sometimes, we might not have enough data, but does that mean that we should not try doing something from fundamentals, is a question. And now, getting into concepts like digital twin, and other things, which are almost becoming an extension of all of AI, where we can tweak in the computer, certain parameters, to see what might happen to, say, a wind turbine on the ... Offshore wind turbines, how will they react to a certain wind, and how do you optimize it in such a way that you get most power out of it? So those are all very interesting concepts.
Brian
So Ajit, you just stepped into an area called digital twinning, and how you can build these models that represent, essentially, reality, and then, it allows you to test these various scenarios, and wind turbines is a great ... Offshore wind is obviously a big investment area for Dominion. Other examples where you've seen those sorts of things? I'm curious, either in implementation inside of your business, or even Paul, if that's even a concept that comes up in some of the teachings and learnings inside of academics.
Ajit
Yeah, digital twin, basically, we are trying to look at usually, rotary elements. They are prone to problems and other things, so it's good to know ahead of time. But one of the classic things which we are still thinking of is outage prediction and other things, using graph methodology, or a digital twin of that, of our circuitry, where it could go, power is out, there are nested outages, how do you find whether it's a nested outage or ... So nested outage is where you bring back power, you think you've fixed it, but then, there's a smaller outage within another area, within the broader area that you've just brought back on power. So all those concepts are very interesting, and digital twins and all can probably help us simulate all those things. And in some ways, we started the journey on AI with CapTech. I mean, you guys kickstarted the whole technology for us, and it's reached a good level of maturity.
Paul
So digital twins, I'd say, as a specific thing, hasn't entered into business education yet. We certainly do build simulations, we try to model reality, and generative AI is having an impact on how we do that.
Jason
Definitely, at the C level, our clients are asking if we shift the language to that idea of simulations and predictions. I mean, what business doesn't want to simulate out their business, and then, project or predict how trends are going to go? And a lot of people are like, can we pull in all these economic factors, and [inaudible 00:11:15]? Well, yes, there are easier things to do in the near term, but the potential of the technology is definitely there, and you'd see every business wanting to probably map that out a little bit.
Ajit
Yeah, now, when you bring that up, it kind of brings me back to the Donald Rumsfeld statement, we have known knowns, known unknowns, and unknown unknowns. So that is kind of what it is.
Brian
Yeah. I want to pull back something, Paul, you said earlier in your first discussion, just around really embracing generative AI, and having students go in and actually try to solve something, but then, it's not working the way they expect it to, so then you have to step back and teach them some of the fundamental aspects of really, how to solve the problem. Not even necessarily leveraging AI, but how to check it. Because that validation part is such a huge piece of how we use AI, and I think it's one of the things that we talk about a lot, is because a lot of people are ... In our industry, you may be fearful that we not going to need computer programming anymore because we can use generative AI to do everything that we've always done. But in reality, I think you just gave one example where that's not necessarily the case.
Paul
They have to validate it, and the first step is getting buy-in, right? I mean, I am terrified for these students, I'm like, you must know how to use this before you go out into the workforce. And we were talking offline about getting the workforce to buy in to the capabilities that these can provide. Understanding the limitations and paying attention to them, I struggle to keep up. Like I said, we run up and down the hall looking at new tools, here and there. I mentioned, we recently had a homework assignment that had a bunch of math notation in it, student tried to use AI, didn't go well. And I happened to find a tool where I could upload my assignment, and it has a little button called explain the math, and it gave all the solutions. So it's a matter of knowing what's out there, what's available, and also, knowing the limitations, and then, being confident that it can help you out.
Jason
Yeah, and the technology is so confident, it gives you an answer-
Paul
Yeah, that's right.
Jason
... the answer to that equation is X. Like, well, no surprise that language models don't end up being very good at math right out of the gate, so you have to use these other kind of mechanisms to get it there. When will it lie? Or ... I'm forgetting the word ... Hallucinate. In certain scenarios, you can't necessarily just believe it at first blush, so it's tricky in that way, because it is very confident.
Paul
And it goes back to the explainability, and that relates to building trust. I think that's going to be a key trend in the next several years, is, how do we increase our trust in these tools, what the output brings, and how do we understand that? So when I first came to Richmond and VCU, I met a professor from another university, and he said, well, what are you working on? I said, well, I'm working on support vector machines. He said, well, I'm working on neural networks, I guess I wasted 25 years of my career.
Jason
Well, he made it [inaudible 00:14:16] early on, right? Hang on.
Paul
Yeah, so for a long time, like I said, optimization and machine learning were kind of synonymous, and there was this opportunity that we could have explainable models and that sort of thing. But now we've got this deep learning and generative AI, it's like this engineering artifact that we can't really explain, so maybe we'll try to get back to capturing some of these fundamental patterns or this behavior, emerging behavior we're seeing, with models that we can understand and explain. And we have to, because I don't see another way to get to the trust issue.
Brian
Trust and verify is just a big aspect of this whole thing, and it comes back to the explainability. And so, I'm curious, in the education space, how are you encouraging people to build trust? How are you encouraging your students to really understand how to develop trust with something that you're developing? And maybe you don't completely understand on the backend, how it all works.
Paul
Like you said, trust but verify. I mean, the moral of the story every night is, oh, look, the AI couldn't solve it at first blush. You're responsible for every line of code, so you need to learn how to verify, how to check it, how to validate it. The challenge for us is, can we teach that without having them do it without AI? Because the paradox is, we can't teach them without them having access to AI, because they have access to AI. So yeah, so that's been a real challenge for us in the higher ed space. I mean, you think about assessments, people are talking about going to oral exams, because you can't have a written exam, can't have take home exams. But I think what we've been focusing on is, like I said, learning how to use a tool, but also, communicate what you've been doing. So that's a real focus for us is, state the objective of the problem, write the results, make me believe that you knew what you were doing, is basically the standard.
Jason
That's good. Some of the AI jargon out there, there's human in the loop, but then, people haven't heard that there's also asleep at the wheel, and it's that kind of too much trust in what it's doing, and that's scary.
Ajit
When you talk about explainability, one classic example was, we had done this 811 analytics solution, where we were kind of looking at the damages that might be caused when people create 811 tickets. Where they're going to be excavating, what is the chance of them digging in and damaging our pipeline? And one of the factors there, from an explainability point of view, was, the factor was, the older the pipeline it is, the more chance that it is going to get damaged if there is excavation close to it. For me, the common sense question I used to always ask, tell me why. And when we started explaining it to the business people, they knew exactly why. They explained the results of our AI on why it is. They said, when the pipelines were placed in underground in early 1900s, we did not have a GIS or a computerized system to record the exact location. So sometimes we kind of have to give the best, most optimal understanding of where the pipeline is, and the damages could occur on it.
So that was explaining to us why certain things were being ranked in a certain way, and it was very interesting to learn. So we kind of do the warning and other things, we have someone who goes and checks, makes sure everything is done. But this was a very interesting tidbit on explainability, so sometimes, when you get together, yeah, we do the analytics, but the people who know the business really can explain some of the results in a better way than we just doing it on our own. So it is the partnership and other things, if you all come together, there's more ... You learn from it. It's very enriching there.
Brian
I'm curious, too, that process, because you talked about really, just the adoption and understanding of these sorts of things. Are you running into a lot of resistance of, there's no way that we could build something that does that, there's no possible way? Are you running into that, and how are you overcoming it?
Ajit
No, I think people have come to terms with AI and the concepts, there's enough in the media now. But initially, we do have to evangelize these capabilities in a sense, because it's such a large organization, how do you take this new thing and let everyone know, and just not know about it, but even understand the nuances of where it could be applied or used? That is where it is, so we do what is called ideation sessions, where we show a few solutions, then we ask them, where do you think it could help? And it kind of builds over there, but it needs a lot of interaction and coming together in person, rather than through Teams or whatever. But yeah, initially, people were a little skeptical because you have experienced people who have been doing the job, how do you convince them? So once they start partnering with you explaining why something is happening, you have a very good following there. I mean, that is the whole part of it, we need to explain how it works. And explainability is a challenge, like we said.
Jason
So you're not threatened by the technology, but you see how it can help you do what you want to do better.
Ajit
Yeah, if it helps them do their job better, they're happy, and it reduces damages and other things, so it's pretty interesting. But they're all boutique AI companies, also, which are doing these kinds of things. So many times, they come do the demo, and we say, we can do that. But that is where you have to be ... Again, we are within the organization, so we have to be making sure that they understand, we have the chops to do it as good as outside vendor specializing in it. But we have to learn, the use cases are interesting once you come to know, hey, they've done this, or we could do that. But to get those ideas is interesting ... I mean, hard, where to apply.
Jason
You both mentioned this a little bit, I'm curious, we talk about doing our jobs better. And a lot of that is, I call it the bottom 20% of our work lives, the stuff that humans shouldn't do. I would be much happier if 20% of my-
Ajit
Jason, you're a modern man, and I agree with you, yeah.
Jason
... I mean, are you running into those now, where like, wow, in two years my students or my coworkers won't have to do X anymore?
Ajit
Yeah, in fact, we have been doing that with robotic process automation. So it takes the repetitive, computer interactive tasks, and it can do it for you. So yeah, as long as there is no subjective aspect to it, and it is something that, it extends into ... After analytics, we are using robotic process automation. For example, from 1900s, we have been having this power distribution set up. And what has happened is, because we did not have everything in the computers, they were all paper-based systems, when they get converted, some of that information won't be accurate, like which meters are connected to which transformer. It's called [inaudible 00:22:00]. We were able to do that as AMI ... Well, I mean, AMI meters were implemented, we could analyze the voltage transition and say, these are the meters which are connected to this transformer. So that kind of things that we come up with on finding these issues and streamlining it is very helpful.
Jason
Little applications everywhere?
Ajit
Yeah, and it all adds to how well the data we collect later on is also more usable.
Jason
Interesting, yeah.
Paul
I just think, also, generative AI just is going to transform all work, not just us technology people, too, right?
Brian
You're speaking my language, Paul.
Paul
It augments the creative processes, and so, you don't have to know machine learning to use AI now. And I think AI is going to be synonymous with generative AI soon, where you need to know the context, you need to be able to talk to the people and understand their problem, and then, we'll ask the generative AI to build the model that we had before, and it's going to do the computation for us.
Brian
It's interesting you say that, because you could make an argument that, until within the last maybe year or so, that when people said AI or machine learning, they were looking at folks like yourselves, folks in the data and advanced analytics space, that are responsible for this sort of thing. But now, it should be something, Paul, to your point, that is prevalent across really, every job, that you're at least considering or thinking how it could possibly make my life better. So I'm curious if you see that actually has transitioned. Who's responsible for AI at VCU in your organization? Is there somebody at Dominion? Is there somebody responsible for it, or what's the philosophy around that?
Ajit
Well, at Dominion, at least, in this case, I am, in some ways, responsible. It was the vision of Steve, our CIO, who's retired. Yeah, we brought in this technology in a big way, and the first few years, went in raising awareness of the capabilities, and now, it is starting to pay the dividends. And it does complement other technologies, like, we find problems, and the data entry in the system has to be changed, you use robotic process automation to go correct the data and the systems. So the synergies of the tools are also coming in together very well. But yeah, I think now, even the businesses constantly looking for these kind of innovative solutions, vendors are knocking on the doors, giving them specific solutions. And it's great, I mean, it's great for the competency of machine learning, AI and others, to have this kind of interest. So I think it bodes well.
Brian
It is amazing how quickly some of the vendor packages and softwares quickly change marketing to say, our solution's built on AI. It was very quick, in the first six months of this year, everything driven by AI, driven by AI.
Ajit
Yeah, smart TV, I mean, it keeps listening to you. You say, play the song, and [inaudible 00:25:10].
Brian
It was always there, and now it's being marketed that way.
Jason
All the vendors this year, coming soon, in 2024. [inaudible 00:25:16], well, okay.
Brian
So I'm curious if you see ... Because you mentioned earlier about you running down the hall and yelling at other professors like, hey, what are you doing in this space? Is there a general consensus that there's somebody that owns the responsibility around AI? Is it the engineers? Is it the physics teams?
Paul
Oh, no, I see it across campus. I mean, the English department hosts AI and the humanities sessions. I see it in the arts, in graphical design. I mean, it's pervasive. And I look with introspection, and I'm like, do I have the right skillset to stay in this these days? What is the skillset now to be able to ... I mean, I can definitely see the value, and the value of your creative mindset is more valuable now, it's gone up, and relative to value of technical skill, I guess, even, a little bit.
Jason
And that's the opportunity, that it's so democratized that, if you're a person now, or if you're an organization, so long as you don't stand still right now, so long as you're moving towards this, then you're in the race. I think that's the biggest thing we've been telling organizations, don't do nothing.
Ajit
What about your AI practice? It must have grown quite a lot, isn't it? In the last six to eight years?
Brian
It absolutely has. And to earlier comments about it somewhat being housed within our data analytics space, just the whole push around generative AI and large language models this year has opened up the conversation to all different applications of what I'll call the traditional or model-driven AI, that people maybe just thought were isolated and siloed in certain areas. Like, oh, well, our data team's got that covered. But now, I think about what ... Maybe I can build a model that does prediction of logistics of where my supply chain needs to put pieces and parts. And that's still not necessarily using generative AI, that's using the same models and stuff we've been doing for a long period of time, but now, people are thinking creatively about how I can use AI in a way that solves a problem that maybe I couldn't solve before.
Ajit
You bring up a very good point, we have this platform for data science, it is called AutoML. And that's pretty good, it kind of figures out what needs to be done on different sets of data, and it comes up with a good result many a time. And we then compare, we do this challenger competition kind of thing, to see which algorithm is better. And our tools give you those capabilities, where you can take four or five different machine learning algorithms and see which one is giving you better results. But with time, that'll be adrift, and then, we need to again run it to see if the same thing is applicable.
Paul
I was going to say, you got to ensure you got the quality data going in first. No leakage, no ...
Ajit
That's true. So it's very interesting how things are happening, in a sense, AutoMLs are generative AI. So soon, maybe we don't need data scientists, in a sense, because if it's going to be all self ... Doing everything in one contained way.
Brian
Well, interesting you bring that up, and I think that ties those two points together, because there's the proliferation of these now curated like specific purpose models, pre-trained models for various purposes. And so, you could ask the question, do we need to have data scientists when we would just leverage one of these existing models in a certain way? But then, you also need to verify data and all the information coming into it. So again, this goes back to the earlier point about, maybe we don't need engineers anymore, or the question about, do we need engineers anymore? The reality is, yes, because you may not have the right model and the right scenario, you may need to look at different data sources or consume data in different ways that you hadn't before, in order to get the outcome that you're looking to get.
Jason
I mean, one of the most refreshing conversations I had a couple of months ago was with, he's the head of the chemistry department at a university. And AI has now figured out all the protein folding. People used to get PhDs in how a protein folds, and we've figured them all out. And I said, does that scare your department, or is that exciting? He said, it's absolutely exciting, we've moved on to the next problem. We've moved on to the next hardest problem. And I think that for any discipline, again, if you can eliminate that bottom 20%, you move on to the next hardest problem. I don't think any, really, line of business or industry needs to be afraid of this if they kind of lean into it.
Ajit
But that is fear, too, in a sense, that is the evil part of AI, also, all the deepfakes and various things that go on. And I think even the federal government is now kind of putting together a team to understand what it might mean, so how AI can be kept for the good and not for the bad. But I think everything is for the good, as well as bad.
Jason
Very important, yeah.
Ajit
Very important to know all tools, starting from the knife, to cut the vegetables, to do other things. So yeah, that's one of the things that we have to understand on, how do you make sure it cannot be misused and cause damage?
Brian
Well, that's an interesting point, because specifically, on the academic space, the AI detection, has this been generated or not, is something that's in the news a lot now, and I think I just saw something yesterday about Meta and Google putting things ... Embedding into their ... Actually, it was Meta, I think, is getting ready to start embedding, January 1, it's going to start embedding in their ads, some sort of watermark about whether or not it was created with generative AI or not. Of course, there's ways to detect that, I'm sure, and avoid that. But I'm curious, is that even a concern? Do we need to detect if this has been generated by AI or not?
Paul
Well, again, I try to impress on the students that you're responsible for every line of code in the end. You're also responsible for the text that you generate, too. So yeah, it's teaching students and our workforce to be careful editors and revisers.
Ajit
That was one of the discussions on NPR, kids using ChatGPT to come up with an essay on various topics, and how are the teachers going to find out whether this was written by the student, or was it a ChatGPT-generated solution? Now, what tools can the teacher use to see if it is a ChatGPT one? Probably give the same question to ChatGPT and get the answers, and see if it matches.
Paul
Similar.
Ajit
Or is it similar? Yeah, exactly.
Paul
Or a follow-up oral exam, that's what we're talking about these days on campus.
Ajit
So yeah, that probably is the way to do it.
Jason
That's funny.
Paul
Can you talk about what you've turned in here? Can you talk about what was produced on paper?
Jason
Did you use ChatGPT to generate this? Well, did you use a car to get to this building today? I mean, it's going to be like ... I don't think that question is going to as be as necessary or informative in the very near future. Well, of course I use the tools that I have available to me to do the best job I can.
Brian
That reminds me of a logic course I took in college, and I remember this, it's not just the resulting of the proof that you got it right, it's all the different translations on the way. It's, show your work example with calculators, and maybe this is just the new version of the calculator.
Ajit
Yeah, one lesser known aspect of the ChatGPT in the general world is the prompt engineering. That is very interesting, too, that you need somebody to prompt and set up prompts in a way that it can give you the right answers. And that was very interesting when we did that research at Dominion, that when we say, give us a list of Dominion holidays, it did not decide that it has to be 2023 holidays, it goes back, and if the data is there, it comes and gives you that list. So the prompt engineering and other things kind of sets the context for that, I mean, the tool to provide you with the right answers. Once they can automate those prompt engineerings based on whatever the question is, then it'll be much more user-friendly, I would think.
Paul
Well, it's that, and also just knowing there's different capabilities and different tools. One can read a PDF, and then answer questions about it. One can highlight math notation, and it can tell you what's going on, explain it in English. But not all of them do that, so it's getting the prompt right, and knowing that there's a tool out there that's got another piece connected to it, that I can upload an image and ask for an analysis of that, or upload a dataset and ask for an analysis of that. And some tools can do that, some tools can't, but it's navigating that. And God knows where we're going to be in a couple of years, where all that's going to converge
Jason:
That's a great point. I mean, we look at tools now, and there's nuances between platforms, and so, you're right, to look at every large ... Oh, it's a large language model, well, you got to have a little bit more sophistication there in the tools. And so, yes, that is worthy work to figure that out.
Brian
Well, you mentioned the next year or two, where that might be, and fearful, maybe, of not really understanding where that might be. But now we're at the pontification phase, where do you see this going? What does that look like two to three years from now, five years from now, whatever time or horizon it is for you, where do you think this is going, inside of your areas, or inside your disciplines?
Paul
Well, I've given a couple hints already. I think, one, we're going to focus on building trust, and trying to evaluate what that means. We're also going to grapple with, what does it mean to be human, and is the computer replicating that or not? I mean, we have tests out there, and that sort of thing, and I'm not well-versed in that, but I think we're going to ...
Brian
You're talking about artificial general intelligence, essentially. Yeah.
Paul
Sure. Yeah, yeah, yeah. We're going to talk about, what does that mean? Or, are we there or not? Yeah, what does it mean? And like I said earlier, I think AI is going to be synonymous with generative AI. Yeah, I mean, we'll still do machine learning, like you said earlier, I think there's a space for the engineers, particularly right now, and explainability, to build that trust and try to find simpler models. But I think because of the way that generative AI is accessible to so many more people, that's going to be the word for AI, is generative AI. That's what they're going to be talking about.
Ajit
In our place, it's more trying to bring about synergies with analytics, bots, and other emerging technology areas, to kind of not have analytics as a separate one, but integrated within business processes, because that is when it is going to be more useful. It's not like the workers in the field are going to now go, I'm going to do analytics now, I'm going to go check. How do you integrate? Earlier, you brought up optimization concept. Long back in engineering, I had studied traveling salesman algorithm and operations research.
So how do you do that, wherein, you know the crew is going to go to the following places? How do you route them in the best way possible? And you could also take the drive times, because it's not as the crow flies. You could also bring in traffic conditions now that you have Google APIs and other things. So all that makes it much more interesting and fun for us. It's fun for us, because we love to dabble in these things, but it's also going to be paying dividends for the company as a whole to have a workforce which is capable. We use it for safety, analyzing telematics data. Safety is big on Dominion Energy's agenda there, so how was the driving habits, what could be done better? All those kinds of things. It's very interesting.
Brian
Well, I'd like to thank Paul and Ajit for joining us today, and Jason, once again, for being a guest on the podcast. And next time, we'll have Vinnie back in behind the mic, and talking about our next topic. But appreciate you all for joining us today, and thank you again for your time.
Ajit
Thank you very much.
Paul
Thanks for having us.
Jason
Thank you guys.
The entire contents and design of this podcast are the property of CapTech, or used by CapTech with permission, and are protected under US and international copyright and trademark laws. Users of this podcast may save and use information contained in it only for personal or other non-commercial, educational purposes. No other use of this podcast may be made without CapTech's prior written permission. CapTech [inaudible 00:37:54] no warranty guarantee or representation as to the accuracy or sufficiency of the information featured in this podcast.
The information, opinions, and recommendations presented in it are for general information only, and any reliance on the information provided in it is done at your own risk. CapTech makes no warranty that this podcast or the server that makes it available is free of viruses, worms, or other elements or codes that manifests contaminating or destructive properties. CapTech expressly disclaims any and all liability or responsibility for any direct, indirect, incidental, or any other damages arising out of any use of, or reference to, reliance on, or inability to use this podcast or the information presented in it.
The entire contents in designing this podcast are the property of CapTech or used by CapTech with permission and are protected under U.S. and International copyright and trademark laws. Users of this podcast may save and use information contained in it only for personal or other non-commercial educational purposes. No other uses of this podcast may be made without CapTech’ s prior written permission. CapTech makes no warranty, guarantee, or representation as to the accuracy or sufficiency of the information featured in this podcast. The information opinions and recommendations presented in it are for general information only. And any reliance on the information provided in it is done at your own risk. CapTech. makes no warranty that this podcast or the server that makes it available is free of viruses, worms, or other elements or codes that manifest contaminating or destructive properties. CapTech expressly disclaims any and all liability or responsibility for any direct, indirect, incidental, or any other damages arising out of any use of, or reference to, reliance on, or inability to use this podcast or the information presented in it.